Convergence in the Digital Era: Why We Believe AI and Web3 Are Poised to Grow [Part 1]
TLDR
The intersection of AI and blockchain is moving fast. From early 2023 through mid-2024, many AI-related tokens have outperformed, highlighting the growing interest in this nascent space.
Much of this hype is driven by speculation, similar to the market dynamic that helped the Metaverse concept explode in 2021.
Despite these frothy conditions, it’s abundantly clear that these two disruptive technologies have the potential to accelerate one another and solve difficult problems. From the democratization of AI to the optimization of blockchain, AIxWeb3 has transformative potential.
In this deep dive report, we explore the AIxWeb3 ecosystem’s infrastructure, middleware, and application layers. We look at investment philosophies, project evaluation frameworks, and emerging trends to explain how we are assessing the space.
As we enter H2 2024, AIxWeb3 keeps growing. This creates space for innovation that will transcend speculation and usher in technological and societal advancement.
Our report covers each layer of the AIxWeb3 stack in depth to explain how to evaluate the space—and why there’s reason to believe in AI and blockchain’s convergence beyond the hype.
The AI Bull Run and How We Got Here
Background
In November 2022, OpenAI unveiled the first version of ChatGPT. The groundbreaking LLM achieved virality on launch, igniting an AI bull run that’s still going strong in 2024. Winners of this run include Nvidia—now the world’s most valuable company— and AI-related tokens like $FET, $RNDR, $TAO, and $AGIX.
In H2 2023 and H1 2024, many teams have been exploring ways to leverage these technologies to break away from the data and computation monopolies held by tech giants like Amazon. AI hype has inspired multiple AI “memecoins”. Yet most projects are either in a conceptual phase or operating Web2 business models that build in superficial tokenomics.
Nonetheless, this market’s speculative nature has sparked interest among retail investors. This interest has been fueled by Nvidia’s continued outperformance, new upgrades to Chat-GPT, and the release of tools like Dream Machine and Sora. With this landscape as the backdrop, AIxWeb3 has become something of a meme akin to the Metaverse in 2021, which briefly surged as future expectations soared ahead of present reality.
This speculative bubble is likely to correct in the future. At Mirana, we are actively seeking out projects and founders that take time to build foundational developments and find genuine ways to leverage crypto and AI.
AI + Web3 = ?
While speculation and incremental advancements have characterized this space’s breakout, the fusion of AI and crypto is more than a series of trendy buzzwords. This convergence could tackle some of the biggest challenges facing both fields today.
Why AI in Crypto?
Merging AI with blockchain isn’t about replacing Web2’s centralized systems but rather enhancing Web3 with decentralized, trustless solutions that foster value creation. There are several ways this can happen:
- Enhancing dApps: By integrating AI, dApps can evolve to resemble Web2 applications, enhancing the user experience and unlocking new possibilities due to Web3’s trustless nature.
- Applications and Use Cases: From improving recommendation algorithms in NFT trading platforms to ensuring fair play with AI opponents in blockchain-based games, onchain AI and its applications will revolutionize efficiency and user engagement.
Why Crypto in AI?
In the realm of AI, blockchain’s decentralized nature can help solve issues such as siloed data and the heavy computational resource requirement for model training.
This technology offers a pathway to democratize the monolithic, Web2-centric AI landscape through collaborative, open-source model training platforms with token incentives.
- Infrastructure Readiness: The ongoing development of infrastructure alongside various scaling improvements in L2 rollups has heralded a breakthrough in Web3’s computing power. This enhancement offers projects the ability to deploy AI on the blockchain instead of relying on traditional, centralized operation models.
- Call for Decentralized AI (DeAI): Individuals and smaller entities increasingly need to leverage sophisticated AI tools and services. This democratization should lead to a plethora of specialized AI models and agents that can perform an array of tasks, interact, and learn from one another in new ways.
As AI evolves, it will require blockchain infrastructure to manage verticals like data privacy, security, and processing power. Conversely, blockchain’s maturation will accelerate the adoption of decentralized applications, enabling more robust, transparent, and secure AI functionalities. Open-source innovation and decentralized infrastructure sit at the core of these developments.
It’s clear that AI and blockchain complement one another and their advancement will lay the foundation for AIxWeb3 solutions to flourish. While AIxWeb3 has benefited from hype, this is not a transient narrative that will fade when market trends change. In fact, we believe that AI and blockchain’s convergence will prove to be extremely disruptive on a technological and societal level.
As we progress, we must make the following assessments:
- What do we genuinely need from AIxWeb3 and what remains a meme?
- Where can AIxWeb3 technologies achieve real adoption beyond crypto narrative?
- Who is building for the long term and who is capitalizing on the hype?
Our Investment Thesis in AIxWeb3
Navigating the Layers
Navigating the AIxWeb3 landscape requires a nuanced understanding of AI, the Machine Learning pipeline, and its various pain points. These include:
- GPU Resource Allocation: Model training requires significant computational resources, which requires powerful GPUs and substantial financial investment.
Pain points: GPU resources are concentrated within large tech companies that can afford the huge costs associated with training large models. Global GPU supply is centralized between Nvidia and AMD, which exacerbates this issue.
- Pre-Training and Data Collection: This layer involves gathering large datasets and preparing them for model training to set the model’s knowledge and skills.
Pain points: This stage is dominated by entities that can access vast data and resources. OpenAI and Google’s extensive data collection and partnerships speak to the issue of centralization here.
- Fine-Tuning and Closed-Source Models: AI models must be refined over time. Their parameters and architecture are adjusted so that they can carry out specific tasks, often within closed-source frameworks that centralize control.
Pain points: Making adjustments in a closed-source manner creates risk of censorship, bias, lack of verifiability, and user privacy concerns. The monopolization of model parameters and deployment strategies among a small number of corporations also presents major ethical and governance issues.
- Inference and Deployment: Trained and fine-tuned models are deployed to make predictions with new, unseen data, bringing AI to end users.
Pain points: Infrastructure for model deployment is centralized on a geographical and corporate level. This poses challenges for accessibility and diversity.
Blockchain can tackle these pain points in several ways. Most projects cover multiple phases in the Machine Learning pipeline, leading us to unbundle it into several layers:
- Infrastructure Layer: This foundational layer focuses on Decentralized Computing (DeComp) and Data.
- Middleware Layer: This layer plays a critical role in Fine-tuning, Inferencing, and Verifiability.
- Application Layer: This layer encompasses Agents and Vertical Use Cases, providing practical applications for AIxWeb3 technologies.

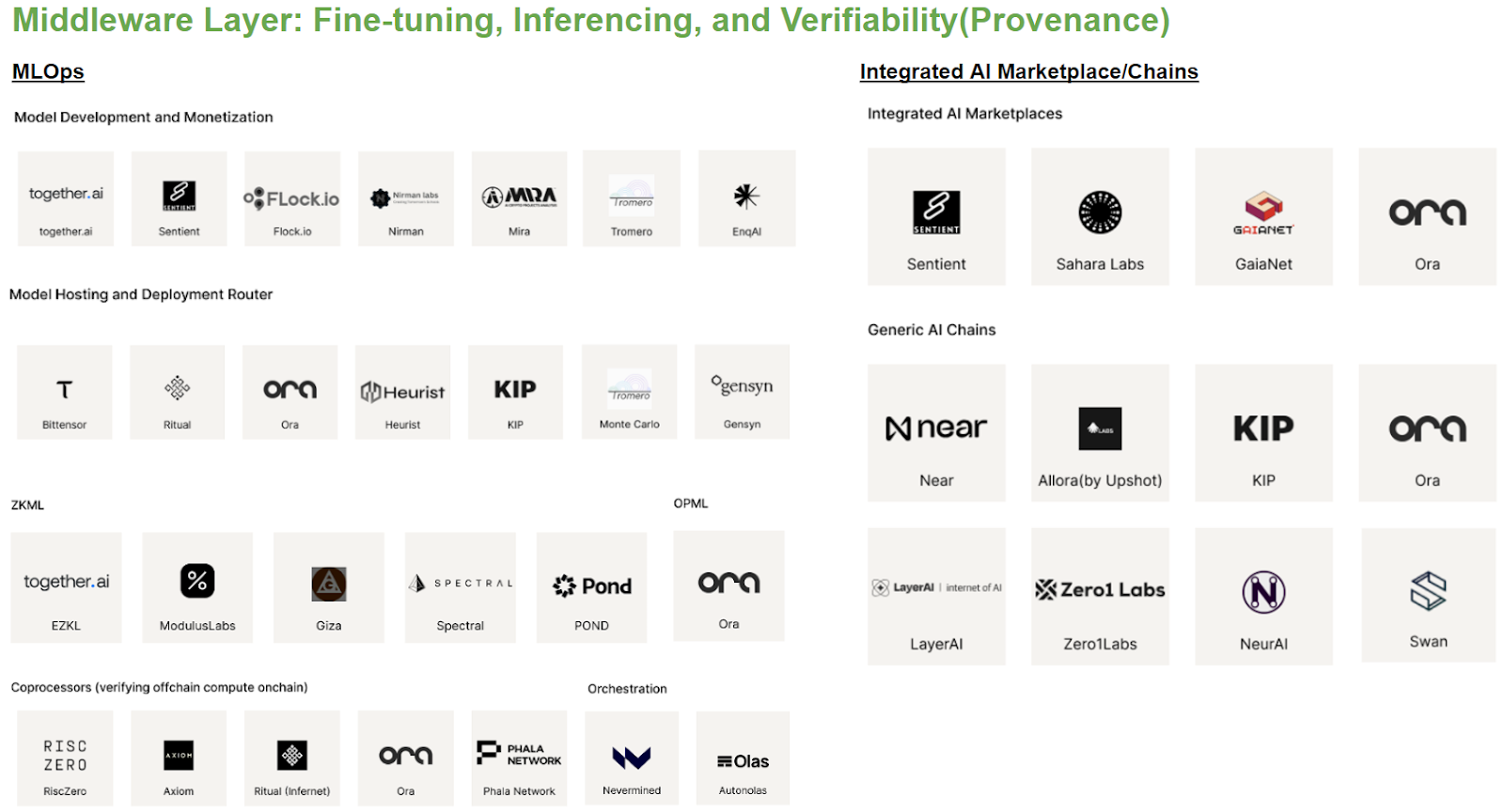
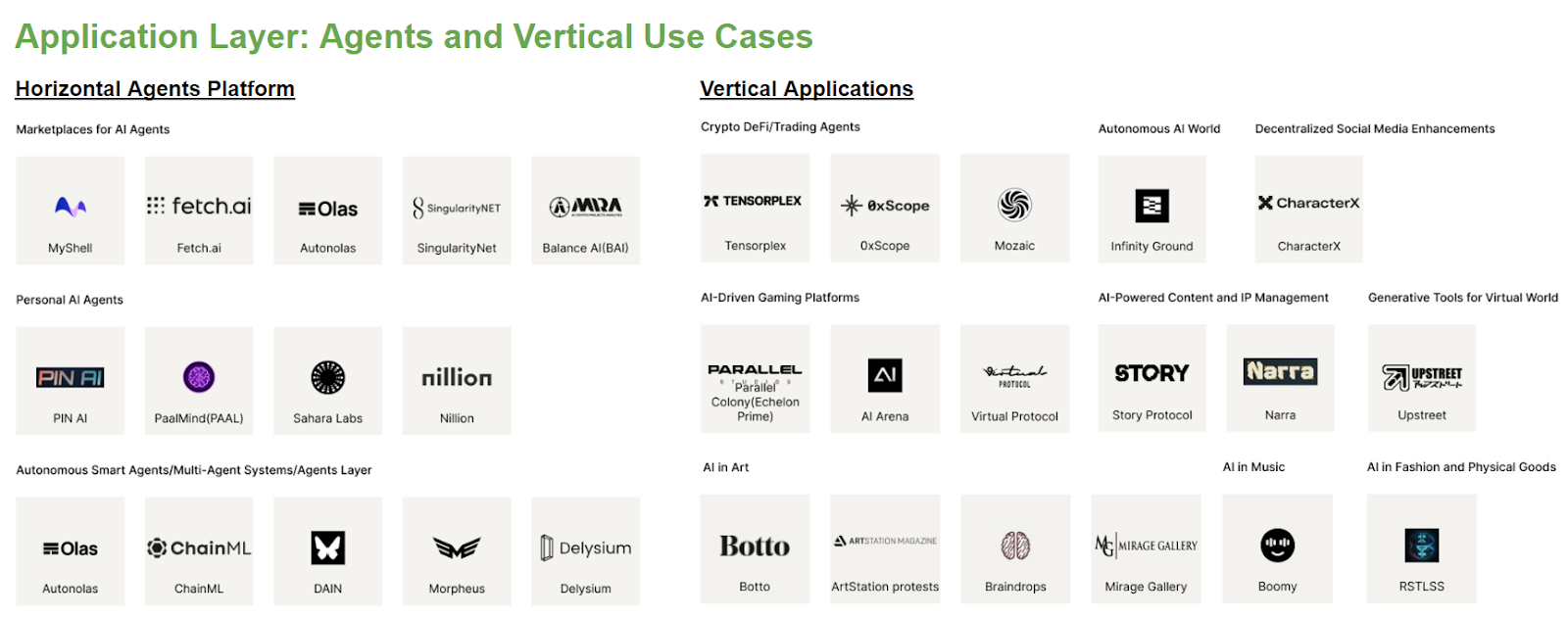
In Part 2 of our report, we will dissect the landscape and analyze key investment considerations for each layer in the stack.
A Framework for Evaluating Projects
Barriers to Entry
- Network Effects and First-Mover Advantage: How significant are the project’s network effects? Do they have a first-mover advantage that could make it difficult for newcomers to gain market share?
- Technology Originality: Is the underlying technology proprietary? Does it offer a unique value proposition or is it just a wrapper for existing solutions? Could a new entrant easily replicate the project’s core technology and market position?
Team and Human Capital
- AI and Crypto Expertise: Does the team have a track record in both AI and blockchain? Do they have sufficient technical and strategic skills to ensure their projects will succeed?
- Network and Business Development (BD) Capabilities: How well-connected are the founders and team within the AI and crypto ecosystems? Could they uncover real use cases and secure end customers for the models or agents developed on their platform?
Viability
- AI Technology vs. Web3 Viability: Does the team understand that using advanced AI technology does not necessarily make the project viable in Web3?
- The Necessity of Blockchain: How important is integrating blockchain technology into this project? Is there a compelling reason to decentralize the project? Could the project’s goals be achieved just as effectively on traditional rails?
- Tokenomics: Is there a rationale behind launching a token? How does the token contribute to the project’s functionality, incentive structure, and economy?
- Product-Market Fit (PMF) and Audience: Has the project achieved PMF in Web3? What factors make this platform more appealing than Web2 alternatives to model or agent developers?
Competitive Edge
- Differentiation Among DeAI Solutions: In the crowded DeAI space, what sets this project apart from its competitors across the infrastructure, middleware, and application layers? Does it offer a unique value proposition?
- User Traction and Client Pipeline: Are there any indicators of user traction or a solid client pipeline that could point to the project's future sustainability? What evidence supports the project’s capacity to grow and retain a user base?
- Showcase Models/Agents: Has the platform successfully developed any showcase models or agents that demonstrate its capabilities and potential for innovation? How do these examples serve as proof points of the platform's value proposition?
Trends and Future Narratives
The AIxWeb3 landscape is rapidly evolving, propelled by significant trends and narratives that shape the technology’s future. Below are some ongoing trends that could impact the space and shape new narratives in AIxWeb3 in the future.
Shift towards Open-Source AI and Democratizing AI Innovation
- Collaborative DeAI Platforms democratize access to AI resources. These platforms leverage blockchain to foster an environment where AI model development, fine-tuning, and inferencing are collective efforts. This trend places focus on community over corporate silos, offering a blueprint for decentralizing AI innovations that breaks down traditional barriers to entry.
- Decentralized cloud services are challenging their centralized alternatives, offering scalable, resilient, and more privacy-centric cloud services for AI applications.
Neural Networks (NNs) & Deep Learning on the Blockchain
- The potential decentralization of AI infrastructure hints at a future in which blockchain platforms host LLMs and neural networks. This could bring greater transparency and security to the space.
- Using crypto to incentivize superior AI outcomes introduces a compelling model for fostering innovation and ensuring equitable reward distribution.
- Onchain Ramps/Oracles for Machine Learning are bridging the gap between offchain AI services and onchain applications, enhancing the ecosystem with rich AI functionalities.
Agentic AI (Agents) and Logical AI
- The evolution of autonomous agents that can execute complex operations and make logical reasoning is enhancing the possibilities of smart contracts and decentralized decision making. This could lead to more autonomous and efficient digital ecosystems.
- Decentralized AI Agents mark a paradigm shift. They enable complex, autonomous interactions in decentralized networks, promising a future of more intelligent and independent digital agents. Custodying AI Agents in a decentralized manner introduces a novel approach that leverages blockchain to ensure the integrity and ownership of agents.
- The integration of AI in DAOs is redefining governance and operational efficiencies, showcasing the potential of AI to contribute to the management and evolution of decentralized organizations.
Multimodal AI
- AI systems are increasingly becoming capable of processing and integrating multiple types of data, such as text, images, and video. This capability enhances applications that use virtual assistants. Such applications can already perform complex tasks like making reservations and planning trips based on multimodal inputs.
Smaller AI Models
- Efficiency and Deployment: We’ve seen the advancements in the development of smaller, more efficient AI models that can be deployed on a variety of hardware. These models are designed to perform complex tasks with limited computational resources. This shift allows for more widespread, practical applications of AI across different devices and platforms.
- Edge AI: Many projects are focusing on the benefits of running AI models at the edge, closer to where data is generated. Edge AI reduces latency, increases data privacy, and decreases the need for constant connectivity to cloud servers. This renders it ideal for applications in autonomous vehicles, smart cities, and IoT devices.
- AI Inference Acceleration: The Triton Inference Server shows how AI inference is accelerating on edge devices. It supports multiple frameworks and optimizes model execution, which is crucial for deploying AI applications in real-world, edge environments.
AI Trust and Safety
- Blockchain solutions can fight deep fakes and misinformation in the digital age. Solutions can enhance the transparency and auditability of AI processes to establish a foundation of trust and safety in digital interactions.
- Validation tools like zkML are becoming essential for maintaining the integrity and reliability of AI within blockchain applications, ensuring that AI actions are verifiable and data is secured.
To Be Continued
As we conclude Part One of our exploration intoAIxWeb3, we have several pressing questions to answer in our next report. In Part 2, we will provide a granular analysis into each of the AIxWeb3 stack’s layers:
1. Infrastructure Layer: DeComp and Data
1.1 Decentralized Computing (DeComp)
- Decentralized General-Purpose GPU
- Decentralized ML-Specific GPU
- GPU Aggregators
1.2 Decentralized Datasets Sourcing and Storage
- Data Availability and Storage Layer
- Data Privacy, Ownership and Knowledge Graph Monetization
- Data Crowdsourcing and Labeling
2. Middleware Layer: Fine-tuning, Inferencing, and Verifiability (Provenance)
2.1 MLOps
- Model Development and Monetization
- Model Hosting and Deployment Router
- zkML
- OPML
- Coprocessors (verifying offchain computation onchain)
- Orchestration
2.2 Integrated AI Marketplace/Chains
- Integrated AI Marketplaces
- Generic AI Chains
3. Application Layer: Agents and Vertical Use Cases
3.1 Horizontal Agents Platform
- Marketplaces for AI Agents
- Personal AI Agents
- Autonomous Smart Agents/Multi-Agent Systems/Agents Layer
3.2 Vertical Applications
- Crypto DeFi/Trading Agents
- AI-Driven Gaming Platforms
- Autonomous AI Worlds
- Decentralized Social Media Enhancements
- AI-Powered Content and IP Management
- Generative Tools for Virtual World Development
- AI in Art
- AI in Music
- AI in Fashion and Physical Goods
What are the pivotal success factors and potential pitfalls within these layers? What criteria do we use to evaluate the vast array of projects across each layer of the AIxWeb3 landscape? What innovative solutions and challenges do these layers present, and how will they shape the trajectory of AI and blockchain's convergence?
Stay tuned for Part 2 of our report, where we will dissect these critical areas and provide an analysis that explains how Mirana plans to strategically engage and invest in the AIxWeb3 space.