Convergence in the Digital Era: Why We Believe AI and Web3 Are Poised to Grow [Part 2]
TLDR
In Part 2 of our exploration into the AIxWeb3 ecosystem, we dive deep into the granular layers shaping this transformative space.
From the foundational Infrastructure Layer, focusing on Decentralized Computing (DeComp) and Data, to the Middleware Layer’s role in Fine-tuning, Inferencing, and Verifiability, and finally to the Application Layer with its Agents and Vertical Use Cases, we explore each sector’s unique challenges and opportunities. This comprehensive analysis aims to arm investors with the insights needed to navigate the complex interplay between AI and blockchain, highlighting the potential for real-world applications and the advancement of AIxWeb3 technologies.
Double Click: Our Investment Thesis in AIxWeb3
In Part 1 of our report, we dissect the landscape by layers. Now, we will deep dive into:
- What are the pivotal success factors and potential pitfalls within these layers?
- What criteria do we use to evaluate the vast array of projects across each layer of the AIxWeb3 landscape?
- What innovative solutions and challenges do these layers present, and how will they shape the trajectory of AI and blockchain’s convergence?
1. Infrastructure Layer: DeComp and Data
The Infrastructure Layer lies at AIxWeb3’s core. It’s critical for providing the computational horsepower and datasets necessary for AI algorithms and decentralized applications. This layer also encompasses DePIN (Decentralized Physical Infrastructure Networks), one of the hottest narratives in crypto today.

1.1 Decentralized Computing (DeComp)
Decentralized computing has emerged in AIxWeb3 in response to severe bottlenecks in GPU capacity. As modern hardware like the NVIDIA H100 Tensor Core GPU is costly and prone to long wait times, startups face a scramble to secure computational resources.
Against this backdrop, various decentralized computing platforms have emerged in hopes of democratizing access to computational resources. They incentivize hardware suppliers with tokens to offer users permissionless clusters at a competitive price range.
Decentralized computing platforms aim to distribute idle computing power across a network, leasing GPUs in the same way Airbnb leases property and Uber leases transportation. This model offers censorship-resistant computing at a lower cost for use cases that can handle latency. However, it faces significant challenges:
- Economies of Scale: Decentralized networks struggle to capture the economies of scale of centralized facilities, especially as the most performant GPUs are rarely consumer-owned.
- Performance: Decentralized computing goes against the grain of performant computing, which traditionally relies on highly integrated and optimized infrastructure setups.
- Technical Bottlenecks: Decentralized networks face significant technical hurdles like data synchronization, network optimization, and data privacy maintenance.
Projects and Categories
- Decentralized General-Purpose GPU: Akash, Render Network, Aethir, Nosana, Fluence, Livepeer, AIOZ, EMC, Koii
- Decentralized ML-Specific GPU: Gensyn, BP-FLAC, Exabit,Tromero
- GPU Aggregators: io.net, together.ai
Key Investment Considerations
- Supply-Side Network Effect: The scalability and decentralization these platforms offer can potentially lower computing costs and increase access.
- Demand-Side Utilization: The success of these platforms hinges on their ability to drive and sustain high utilization rates, which requires strong business development strategies.
- Creative Tokenomics: These platforms will be able to build robust ecosystems if they use innovative token economics strategies to incentivize resource providers and users.
Our Conclusions
There is clear demand for accessible, cost-effective computing resources, particularly among smaller entities that cannot compete with big corporations. This illustrates the value proposition of decentralized computing networks.
But while Web3 offers transparency, cost reduction, and efficiency, it does not fundamentally disrupt traditional network operations or solve technical bottlenecks. This leads us to the following conclusions:
- Projects that address specific market needs—such as cost-effective solutions for batch processing tasks that are less dependent on immediate, high-speed computation—have a higher potential for adoption.
- Decentralization should be used as a means of reducing costs through disruptive network models and token designs.
- Successful decentralized computing networks will align with user needs, offering economically attractive solutions that go beyond providing computational resources.
In summary, projects must demonstrate that they can offer sustainable, cost-effective solutions to meet real-world demands, balancing innovation in token design with scalability and practicality.
1.2 Decentralized Datasets Sourcing and Storage
Data forms the backbone of all AI models. As a result, data is potentially the key differentiator in the performance of specialized AI applications. Datasets are increasingly becoming monetized, as evidenced by partnerships like Reddit’s $60 million licensing deal with Google.
Blockchain’s ability to offer token incentives could pave the way for the creation of industry-specific datasets and private personal datsets while preserving privacy of datasets contributors through cryptographic technologies.
Projects and Categories
- Data Availability and Storage Layer: Zero Gravity, AO (Arweave), Glacier Network, RSS3, Synternet, Subsquid (SQD), Filecoin, IPFS
- Data Privacy, Ownership and Knowledge Graph Monetization: Sahara Labs, Nillion, Ocean Protocol, Privasea, ModulusLabs, OriginTrail, 0x0Exchange
- Data Crowdsourcing and Labelling: Grass, Openlayer, AIT Protocol, Synesis One, Web3Go (DIN), PublicAI
Key Investment Considerations
- Data Quality and Diversity: Access to valuable private domain data is a key successful advantage that leads to monetization. Platforms are more likely to succeed if they implement mechanisms to verify and maintain data quality through Community-driven validation processes and incentives.
- Scalability and Cost-Efficiency: Platforms that can scale by reducing the costs associated with storage and transactions without compromising performance will likely lead the market.
- Regulatory Compliance and Data Privacy: Platforms that integrate privacy-preserving technologies like Zero-Knowledge Proofs could offer significant competitive advantages.
- Tokenomics and Incentive Structures: Platforms should ideally adopt token models that align incentives among all stakeholders, including data providers, consumers, and validators. Platforms that provide clear, direct benefits for contributing high-quality data and maintaining network integrity are more likely to succeed.
- User Adoption and Network Growth: Platforms have greater adoption potential if they implement considered strategies to focus on user acquisition and retention, effective community management, partnerships, and marketing.
- Data Portability and Interoperability: Platforms that support standards and protocols for easy data portability and interoperability with other systems are likely to be more attractive to users.
Our Conclusions
AIxWeb3 needs diverse proprietary datasets. The centralized nature of today’s large datasets proves this. Decentralized data sourcing could mitigate the risk of monopolistic control and improve model training diversity. All of the following points are clear:
- The key value proposition of data crowdsourcing platforms lies in their ability to get sustainable and valuable datasets from private domains. This requires an effective incentive mechanism supported by solid demand from clients to provide sustainable economic rewards. Active user participation and network effects leads to success.
- The adoption of decentralized dataset sourcing and storage depends on the development of robust, standardized interfaces that can compete with centralized marketplaces.
- Decentralized networks must handle large volumes of data efficiently to compete with centralized services. Cost efficiency is also key for user acquisition and retention. Projects will need to be able to move data seamlessly across platforms as the digital ecosystem becomes more interconnected.
In summary, projects must prove that they can provide secure, scalable and efficient data solutions that cater to the evolving demands of data-rich applications. Projects must balance innovation in data handling and tokenomics with robust privacy protections and seamless interoperability.
2. Middleware Layer: Fine-tuning, Inferencing, and Verifiability (Provenance)
Middleware connects raw computational power to end user applications. This layer is crucial for the development and deployment of AI models. Integrating token incentives to reward each value creators along the machine learning workflow, including GPU suppliers, datasets contributors, model developers (pre-training/fine-tuning/inferencing), and validators, offers transformative potential by allowing fractional ownership of AI assets, improving liquidity and offering unique investment opportunities.
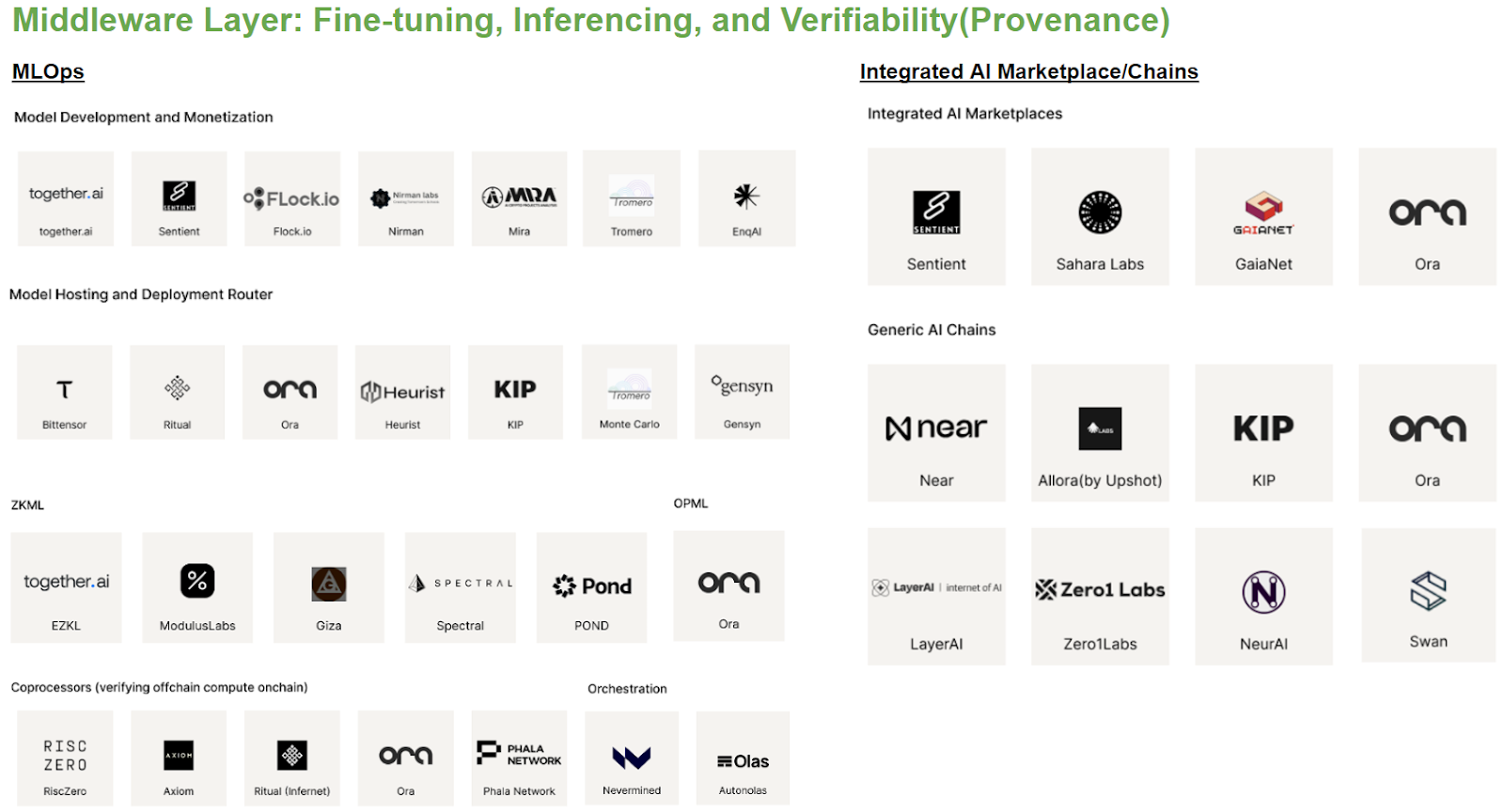
2.1 MLOps
Integrating token incentives to reward each stakeholders (GPU supplier, datasets contributor, model trainer, validator) in the machine learning workflow offers transformative potential in a variety of ways:
- Fine-tuning Phase: Projects can use tokens to reward contributors for optimizing weights, fine-tuning, and participating in RLHF (Reinforcement Learning with Human Feedback), then drive advancements in AI model quality. Projects generally offer APIs/SDKs similar to a PaaS platform to facilitate the model training process.
- Inferencing Phase: The deployment platform delivers the inference to the end user via on-premise servers, cloud infrastructure, or edge devices. Inferencing with ZKML and OPML serves as the gateway to bringing ML onchain, enabling scalable AI and ML capabilities for Ethereum, all while maintaining decentralization and verifiability.some text
- Trustless inferencing, particularly through technologies like Zero-Knowledge Proofs (ZKPs) and Optimistic Proofs (OPs), offers a way to validate the authenticity of model outputs without revealing the underlying data or model specifics. Onchain model verifiability will unlock composability, offering a way to leverage the output across DeFi and crypto. Model verifiability is akin to reputation for intelligence.
- AI Orchestration: To enable generic AI operations together with omnichain access on blockchain and build on-chain AI.
There are several key considerations from studying the middleware layer focusing on MLOps:
- There are many vaporware projects in this layer telling identical narratives. This means it’s crucial to identifyteamsthat are building for the long-term versus those capitalizing on the hype.
- Integrating crypto incentives does not guarantee high standards in MLOps, where precision and quality are crucial. The ability to develop proprietary models, easy to use orchestration tools and add value to developers with deep AI know-how is key.
- Onchain models are not a must-have. AI models do not necessarily need to live onchain to operate trustlessly. Most users are more concerned with satisfactory outputs than the trustlessness or transparency of the inferencing process.
- Verifiability is not fundamental. Users typically do not verify the models they run; they are more interested in the results than the process. This reflects a misalignment between the promise of Zero-Knowledge technology and user concerns.
- Open-source models could suffer from demand challenges as they decrease in size. While larger LLMs cannot run on lightweight devices like smartphones, high-performance desktop computers can handle these models.
Projects and Categories
- Model Development and Monetization: Together.ai, Sentient, Flock.io, Nirrman, Mira, Tromero, EnqAI
- Model Hosting and Deployment Router: Bittensor, Ritual, Ora, Heurist, KIP, Monte Carlo, Gensyn
- ZKML: EZKL, Modulus Labs, Giza, Spectral, POND
- OPML: HyperOracle, ORA
- Coprocessors (verifying offchain computation onchain): RiscZero, Axiom, Ritual (Infernet), ORA (OAO: Onchain AI Oracle), Phala Network
- Orchestration: Nevermined, Autonolas
Key Investment Considerations
- Intellectual Property and Technical Moat: The strength of proprietary technology, access to specialized models, private datasets, and algorithms are all factors that can help platforms beat out their competitors.
- Developer tooling/SDKs/APIs for blockchain and application integration: Platforms have a better chance of success if equipped with tools for model customization and support for AI frameworks.
- Community and Ecosystem: Platforms are more likely to thrive if they can foster a vibrant community of developers, users, partners that contribute to the ecosystem.
- Tokenomics and Incentive Structures: Platforms can use economic models to incentivize participation and ensure sustainability. The most successful projects will be those that use these structures to acquire users and go to market, driving monetization and rewarding contributors.
- Network Effects: As the value of marketplaces grows when they attract more users, building large and active user bases is crucial.
- Monetization Channels: While challenging, platforms must find effective ways to monetize. Implementing clever tokenomics models plays a crucial role in incentivizing creators and users.
- AI Integration and Interoperability: Projects will need to offer seamless integration across multiple ecosystems to gain traction in the cross-chain era.
Our Conclusions
- Token incentives require consideration and workflow optimization: Token incentives can drive significant advancements in AI model co-development by rewarding contributions to model training and fine-tuning. However, they must be carefully designed to ensure high standards in MLOps, where precision and quality are paramount.
- Decentralizing infrastructure is difficult:Decentralized inferencing faces significant infrastructure challenges. Integrating Zero-Knowledge Proofs (ZKPs) or Optimistic Proofs (OPs) for trustless inferencing adds complexity but offers a pathway to scalable, onchain AI that maintains decentralization and verifiability.
- Verifiability is not a must-have: Users prioritize effective AI outputs over the transparency or trustlessness of the inferencing process.Therefore, while onchain verifiability and trustless operations are innovative, they are not fundamental to user satisfaction.
- Open-source models have limitations: While open-source models are beneficial for transparency and collaboration, they may struggle with demand challenges as model size decreases. The ability to handle large models on lightweight devices remains limited.
In summary, projects in the MLOps middleware layer must balance innovation in token incentives and onchain verifiability use cases to provide high-quality, sustainable AI solutions. Investing successfully in this layer requires identifying teams dedicated to long-term development rather than short-term hype. Teams must have proprietary models, robust orchestration tools, and deep AI expertise to create value in this space.
2.2 Integrated AI Marketplace/Chains
The term “AI Marketplace” represents more than just a trading platform for AI models. In fact, AI marketplaces are fully financialized middleware that span the entire AI supply chain. This includes computational power, data, AI models, and their applications. AI marketplaces act as entire ecosystems with many monetizable components. In Web3, a successful AI marketplace could potentially generate demand for decentralized computing platforms, which currently suffer from oversupply and low demand.
- Model Marketplaces: IMO (Initial Model Offering) and model marketplaces on top of model development platforms. Such a marketplace would cater to the creation of demand for decentralized computational resources and foster a closed-loop system where AI’s full lifecycle is monetized at once.some text
- Investment in AI Models: When AI models are investable assets, users can directly participate in the technology’s value creation process. Revenue sharing models ensure that contributors reap financial benefits proportional to the performance and application of their models.
- AI Chains: A modular layer specifically built for AI apps, where models and data owners can build, transact, and monetize in Web3.
Projects and Categories
- Integrated AI Marketplaces: Sentient, Sahara, GaiaNet, ORA
- Generic AI Chains: Near, Allora (by Upshot), KIP, ORA, Swan, LayerAI, Zero1Labs, NeurAI
Key Investment Considerations
- Market and User Adoption: The success of these AI marketplaces will hinge on their ability to meet the specific needs of developers and users. Those that answer to user needs are more likely to achieve meaningful adoption.
- Innovation and Differentiation: AI marketplaces must continuously innovate and distinguish themselves from centralized platforms. They should offer unique advantages such as superior data diversity and attractive economic incentives.
- The Quality of Models and Applications: The core ofa platform’s value proposition lies in the quality of the AI models it hosts. High-quality, effective models are essential for attracting both developers and end users.
- Community and Ecosystem Growth: Building a robust, active community is crucial. The platform must foster a conducive environment for collaboration and innovation, ensuring that community contributions are valued and rewarded.
- Intellectual Property and Technical Moat: AI marketplaces that have robust proprietary technology, access to specialized models, private datasets, and algorithms will be well-positioned to take a lead against their competitors.
- Developer tooling/SDKs/APIs for blockchain and application integration: Platforms should ensure that they have tools for model customization and support for AI frameworks.
- Tokenomics and Incentive Structures: Platforms must employ effective economic models to incentivize participation and ensure sustainability. Platforms should use incentive mechanisms to acquire users and go to market, driving monetization and rewarding contributors.
- Network Effects:Marketplaces grow in value as they attract more participants, so they should focus on building large, active user bases.
Our Conclusions
- AI platforms must answer to the market’s needs: The success of AI marketplaces hinges on their ability to meet the specific needs of developers and users. The core value proposition of AI marketplaces lies in the quality of the AI models they host. High-quality, effective models are essential for attracting developers and end users, ensuring the platform’s credibility and utility. Platforms that effectively cater to user demands and provide valuable tools and resources are more likely to achieve widespread adoption.
- Innovation and differentiation is vital: Continuous innovation and differentiation from centralized platforms are critical. AI marketplaces must offer unique advantages such as superior data diversity, attractive economic incentives, and seamless integration with decentralized computing resources.
- Building an active community is a must: Building a robust, active community is crucial for sustained success. Platforms must foster a collaborative environment that values and rewards community contributions, driving innovation and engagement.
- Intellectual property can act as a technical moat: AI marketplaces with strong proprietary technology, access to specialized models, private datasets, and advanced algorithms will have a significant competitive edge, positioning them as leaders in the space.
- Platforms must provide developer tooling: Ensuring that platforms offer comprehensive tools for model customization and support for various AI frameworks is vital. This will enhance the platform’s usability and attract a broader developer base.
- Tokenomics and incentives are key: Effective economic models are essential for incentivizing participation and ensuring the platform’s sustainability. Platforms should implement incentive mechanisms that drive user acquisition, market penetration, and monetization, while rewarding contributors fairly.
- Winners must attract loyal users: As AI marketplaces grow, they must focus on building large, active user bases. The value of the marketplace increases with the number of participants, making network effect a crucial factor in achieving long-term success.
In summary, AI marketplaces must balance continuous innovation, high-quality model offerings, robust community growth, and effective incentive structures to provide sustainable, differentiated, and valuable ecosystems that cater to the evolving demands of developers and users.
3. Application Layer: Agents and Vertical Use Cases
AIxWeb3’s potential will be realized at the application layer. This is where users can access direct interfaces and services across various domains.
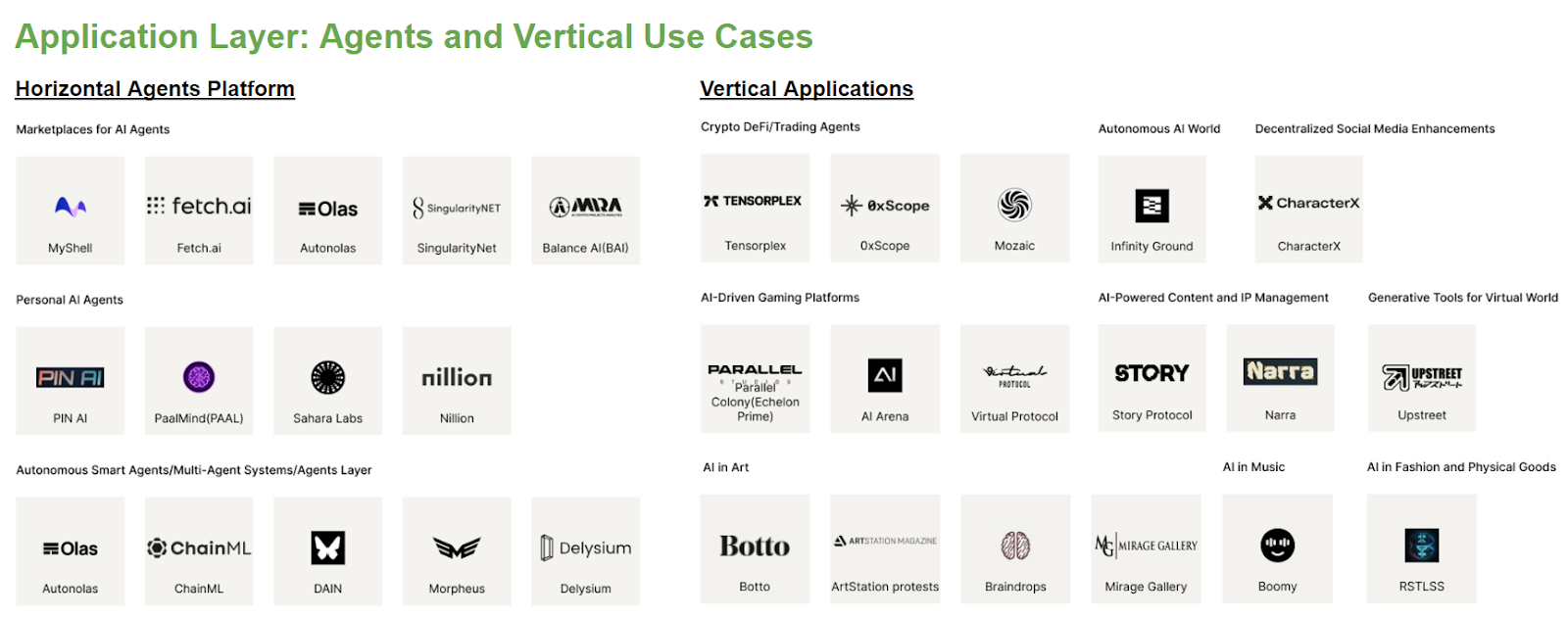
3.1 Horizontal Agents Platform
It’s widely expected that AI agents will experience growth in their capabilities and adoption. Over time, they will become more capable of executing complex tasks in an autonomous manner. Autonomous AI agents are becoming more prevalent. These agents can execute tasks with minimal human intervention with the support of permissionless, trustless blockchains.
The trends towards smaller AI models and highly performant AI chips on Edge devices (such as the Apple M4 chip) lead to more promising personal AI agents with on-device LLMs.
AI marketplaces and tokenization platforms are transforming the AIxWeb3 landscape by creating avenues for trading AI models and services. These platforms are increasingly commodifying and financializing AI assets.
Projects and Categories
- Marketplaces for AI Agents: MyShell, Fetch.ai, Autonolas, SingularityNet, Balance AI (BAI)
- Personal AI Agents: PIN AI, PaalMind (PAAL), Sahara, Nillion
- Autonomous Smart Agents/Multi-Agent Systems/Agents Layer: Autonolas, ChainML, DAIN, Morpheus, Delysium
Key Investment Considerations
- Market Adoption and User Interface: The success of AI agent platforms will depend on their ability to simplify complex blockchain interactions. Projects that focus on offering users a simple interface and smooth user experience to increase adoption are likely to win in the long term.
- Technological Innovation and Differentiation: Many similar platforms are in close competition, so innovation that differentiates one from another is key. Unique features like advanced natural language processing capabilities and integrating with existing blockchain infrastructure could give projects a competitive edge.
- Ecosystem Development: Platforms are more likely to nurture a vibrant ecosystem if they focus on their developer and user communities. Platforms that provide tooling and developer support to create and deploy agents or incentivize user-generated content and interaction have a better chance at attracting developers and users.
- Partnerships and Network Effects: Agent platforms can enhance their functionality and reach by forging strong partnerships with other blockchain projects, data providers, and financial institutions. Platforms that effectively leverage network effects can rapidly scale and capture market share.
- Longevity and Vision: The most promising projects have a long-term vision and the potential to adapt to future technological advancements. Platforms that implement a clear, adaptable long-term strategy are more likely to navigate the evolving landscape of AI and blockchain technology successfully.
Our Conclusions
We can draw several conclusions from our research into AI agents. They can be summarized as follows:
- While AI agents are just emerging, they are improving: AI agents are only just emerging as projects begin to deploy them to execute simple tasks. But these agents will become more autonomous over time. They could offer improvements in user experience across all crypto verticals, helping transactions evolve from point-and-click to text-based interactions via LLMs.
- AI assets are likely to become more commodified and financialized: The rise of marketplaces for AI models and tokenization platforms hints at increasing commodification and financialization of AI assets. These assets will live on top of blockchains.
- Crypto rails can benefit agents: Crypto rails are appealing for agent transactions as there are no chargebacks and they can process microtransactions. Today, agents are most effective for crypto-to-crypto transactions, but they could become more sophisticated over time.
- Agents need to evolve as user demands become more complex: If agents are to execute more complex tasks, new developments in models and tooling are key. Crypto must also achieve regulatory clarity and broader adoption as a payment form for agents to become useful to regular people.
- Patience and long-term time horizons are crucial: The practical deployment of effective multi-agent systems is likely only possible on a longer time horizon, potentially requiring new level-ups in AI and blockchain technologies. Agents are likely to become some of the biggest consumers of decentralized computing power and Zero-Knowledge Machine Learning (zkML) solutions. In the long term, they may be able to act in an autonomous and non-deterministic manner to receive and solve any task.
Insummary, AI agents will significantly enhance user experiences across crypto verticals by evolving from simple task execution to sophisticated, autonomous operations. In the future, we are optimistic about a multi-agent systems powered by crypto payments,even though this could take a long time to achieve.
3.2 Vertical Applications
Sector-specific applications such as Parallel Colony (gaming) and CharacterX (decentralized social media) illustrate how AI can enhance user experiences and operational dynamics across different niches. These applications prove that integrating AI will not only be beneficial for technological adoption.
The most successful applications will likely integrate AI and adapt and evolve to meet their users’ changing needs and expectations.
Projects and Categories
- Crypto DeFi/Trading Agents: Tensorplex, 0xScope, Mozaic
- AI-Driven Gaming Platforms: Parallel Colony (Echelon Prime), AI Arena, Virtual Protocol
- Autonomous AI World: Infinity Ground, Stanford AI town
- Decentralized Social Media Enhancements: CharacterX
- AI-Powered Content and IP Management: Story Protocol, Narra
- Generative Tools: Upstreet, Today
- AI in Art: Botto, ArtStation protests, Braindrops, Mirage Gallery
- AI in Music: Boomy
- AI in Fashion and Physical Goods: RSTLSS
Our Conclusions
We’ve made the following assessments from researching some of the leading applications on the market today:
- AI is often a complementary technology, not a core technology, in Web3 vertical dApps. Projects that integrate AI to augment their core competencies often realize practical, immediate benefits.
- Many application layer projects have integrated AI, but few projects have used the technology in meaningful ways. Many integrations are using AI for only basic enhancements today.
- There is a vision for a self-sustaining world where AI agents interact with one another autonomously. However, this is a long-term view. A world like this requires dynamic AI-powered interactions, offering a deeper level of engagement and complexity.
In summary, vertical application investment is still heavily reliant on domain-specific expertise rather than purely on AI technology. However, we look forward to seeing more projects building native AI applications, such as a self-sustaining world where AI agents interact autonomously in games or social environments.
To Be Continued
In our upcoming research reports, we will publish further deep dive research into several promising AI projects we’ve been following closely. Please stay tuned and subscribe!